Experiments
Process Mining to Enhance Maintenance Service Logistics (PROMAS)
ITALY, “Veneto” region
SMACT
Experiment objective
-
Maintenance service logistics encompasses all processes that ensure that the resources required for maintenance are at the right place at the right time. In a broader sense, it also includes maintenance planning and design-for-maintenance. The objective of this experiment is to enhance and optimized maintenance service logistics of production equipment/machine tools through AI-powered process mining techniques. Process mining is the deep-dive analysis, discovery, monitoring, and improvement of as-is processes, revealing the to-be efficiencies your business benefits from. It provides insight on potential improvement, focusing on finding better, more efficient pathways in process workflow to minimize costs, time and human intervention. Within the context of PROMAS experiment application of process mining methodology to the maintenance service logistics will result in models describing the overall processes of manufacturing organizations from the open of a maintenance call till its successful closure, in order to identify bottlenecks, to compare event logs with prior model(s) to understand whether the observations conform to a prescriptive or descriptive model.
This experiment will be carried out at Treccani Engineering premises, located in Verona, Italy. The DIH of the experiment is “SMACT” that is located in Venice, Italy. Both organizations are based in the Italian region “Veneto”.
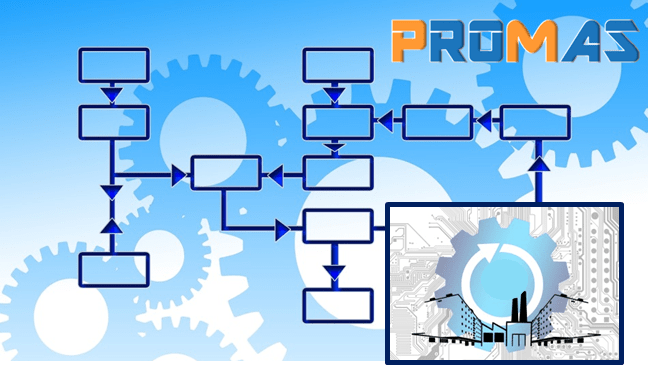
Challenges
- Challenge 1: Extracting and preparing the proper data. One of the first insights when starting to look for process mining data is often that there are data quality problems. This insight is already valuable by itself, because the data is often also used for other types of analyses. Extracting and preparing the data can take time in the beginning, but it gets easier over time.
- Challenge 2: Finding/selecting the right algorithms for process mining. Algorithms have a big impact on results and must be carefully selected/implemented according to the actual scenario and scope
- Challenge 3: Maximizing not just productivity – but resiliency. Identifying and resolving all bottlenecks and noncompliance issues. Adopting solutions that are robust as possible against process noise and uncertainty.
Implementation of the Solution
Every time a process is completed, it creates data. For example, any time a customer service request is received, you know when the call was received, who handled it, how long it took, whether the issue was resolved, just to name a few. It could also include time-stamped logs of purchases made at a register. This generates a so called ‘event log’ files store in an ICT systems. Currently such dataset are parsed by simple analytics tools to understand some general trends – how long things take, the total volume of processes completed, who is handling what, etc. This is where a AI-powered process mining solution of PROMAS comes in. It provides novel insights that can be used to identify the executional path taken by operational processes and address their performance and compliance problems. The solution will be exploited as a digital service for all customers (manufacturing companies) that need to enhance and optimizes their maintenance service logistics processes. Process Mining solution includes three main components:
- Process Discovery: Process Discovery identifies the business processes, maps and analyzes an organization’s existing business processes, uncovers the process steps that they are not aware of, and realizes process deviations. A discovery technique takes an event log and produces a process model without using any prior information. The following algorithms will be investigated, and the best performing will be implemented: Alpha miner, heuristic miner, DFG miner, IFP miner, inductive miner.
- Conformance checking: is a technique to compare a process model of the actual process with an event log of SOP (Standard Operating Procedures) defined by the company. The goal is to check if the event log conforms to the model and vice versa. The model derived from process mining is compared with the perceived process model based on SOP. To perform the comparison, a Business Process Modell Baseline (BPMB) is created based on the specific SOP and fed in to the PROMAS process mining tool to identify deviations in factors such as path, time and cost.
Enhancement: The idea is to extend or improve an existing process model using information about the actual process recorded in the event log. This process has the capability aims at changing or extending the existing model.
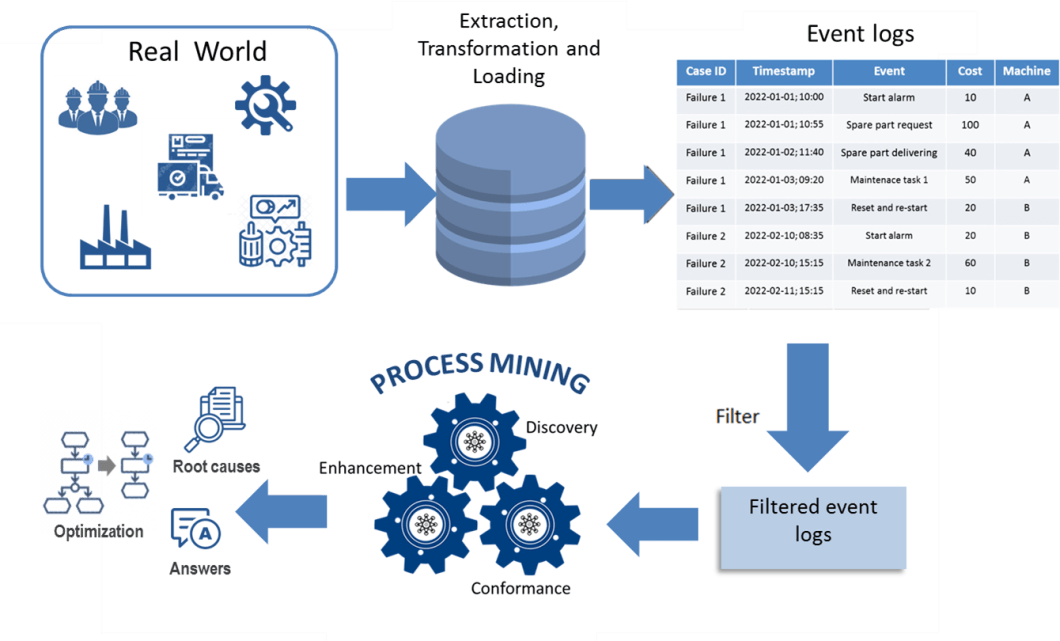
Dissemination
Logo of the experiment:
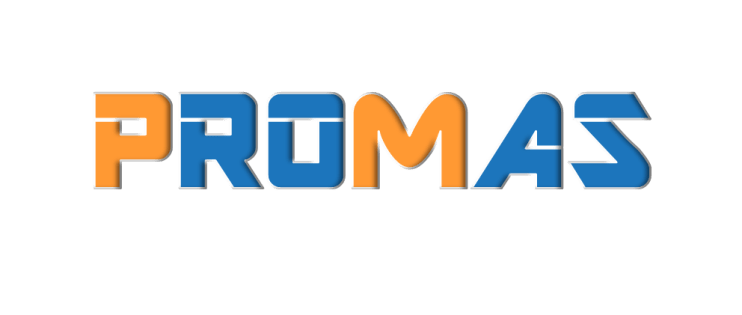
Web page of the experiment: https://www.smact.cc/progetto-promas
Project flyer: https://www.smact.cc/_files/ugd/476fed_1002ce1c29aa4f439ee68e6a76317911.pdf
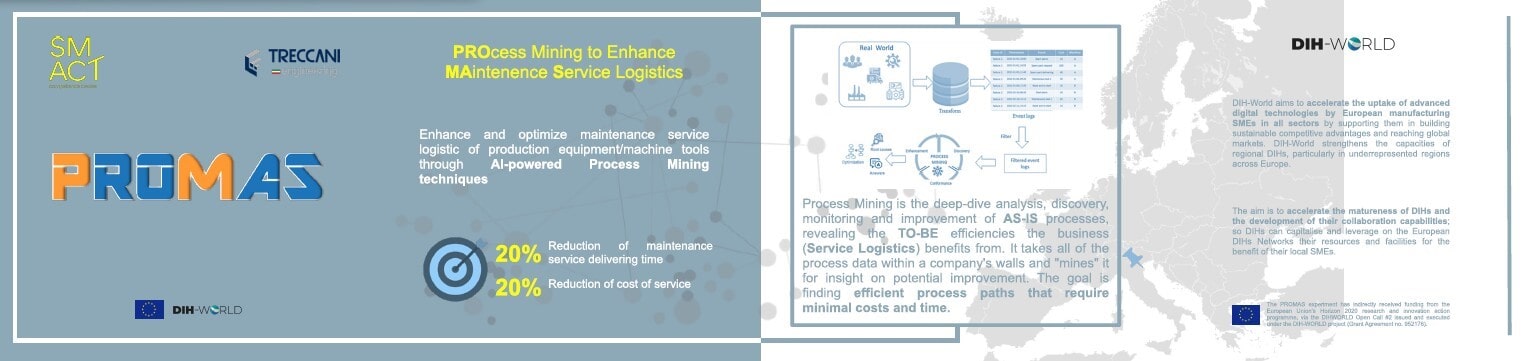
LinkedIN posts: