Experiments
PROOFER – deePleaRning mOdels fOr FootwEaR
Italy, Lombardy
ComoNExT – Innovation Hub
Experiment objective
The objective of the experiment is to develop an automatic and digitalised quality control system for the footwear industry by using an innovative artificial intelligence (AI) logic, customised for this sector. In footwear, the final product should not present any kind of defect such as nails, staples, or metallic parts which are not structural or necessary, and which might hurt the final customer. Moreover, a pair of shoes delivered to the final customer should be of the same size and model. No error is allowed. PROOFER will make use of high quality, last generation X-Ray scanners to acquire shoes images, which in turn are analysed by a computer vision (CV) system, equipped by a deep learning (DL) model trained to recognize the desired defects. The goal is to reach a performance suitable for industrial applications. By implementing this experiment Brustia will own a technology with no equals in the footwear industry, which will significantly improve the company image. Thus, Brustia will be able to propose customers an innovative and unique machine, which promises to increase the sales. All the shoes’ producers who will decide to buy the machine will also experience several benefits. A completely automatic and unsupervised quality control will free human resources for higher level tasks, and thus reduce costs. Moreover, the industrial processes will gain effectiveness and the product quality will enhance. Indeed, even if a machine cannot guarantee 100% of performance in the quality check, it can assure better performance than a human operator. |
Challenges
Since this experiment lies at the border between industrial applications and pure research, the challenges are numerous.
- 1st The objects to identify are small, with a not defined shape and a not constant contrast. The variability of these objects is high. Therefore, the problem is difficult to tackle from the scientific point of view. For this reason, in this experiment very advanced techniques and methods for object recognition have been implemented by taking inspiration from other fields of application, such as satellite image analysis.
- 2nd Another difficulty is related to performances. In industrial application errors must be limited to the minimum. For each not detected defect remained into the shoe, which may hurt the final customer, the producer must pay a penalty. Therefore, the performance must be very high and guaranteed in different environments, that are the production lines of many different shoes’ producers. For this reason, in this project many highly innovative DL models have been implemented, customised, set and tuned to find the best solution and guarantee the best performance.
- 3rd To reach the desired performance there is no secret: we need big amounts of data. This constitutes another difficulty, since acquiring, collecting, and especially annotating many images implies to spend a tremendous amount of time. For this reason, this project aims at building an ecosystem composed by the Brustia’s customers which can provide big amounts of data in a common, centralised database managed by Brustia. The shared data will contribute to improve the performance of the DL model, that in turns can be updated for all the customers who can take advantage of a system which becomes more and more efficient and errorless. Easy data availability is the cornerstone of the success of any machine learning project.
- 4th Last challenge is related to the management of the experiment. Time is critical in such a project. Within the project lifespan, we will have to collect and annotate tens of thousands of images. Human resources’ time is a very scarce and valuable resource, nevertheless the grant obtained thanks to this 2nd open call will allow Brustia to dedicate 3 people in its R&D team to the project implementation.
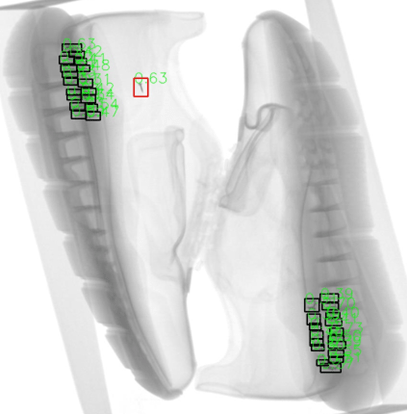
Implementation Solution
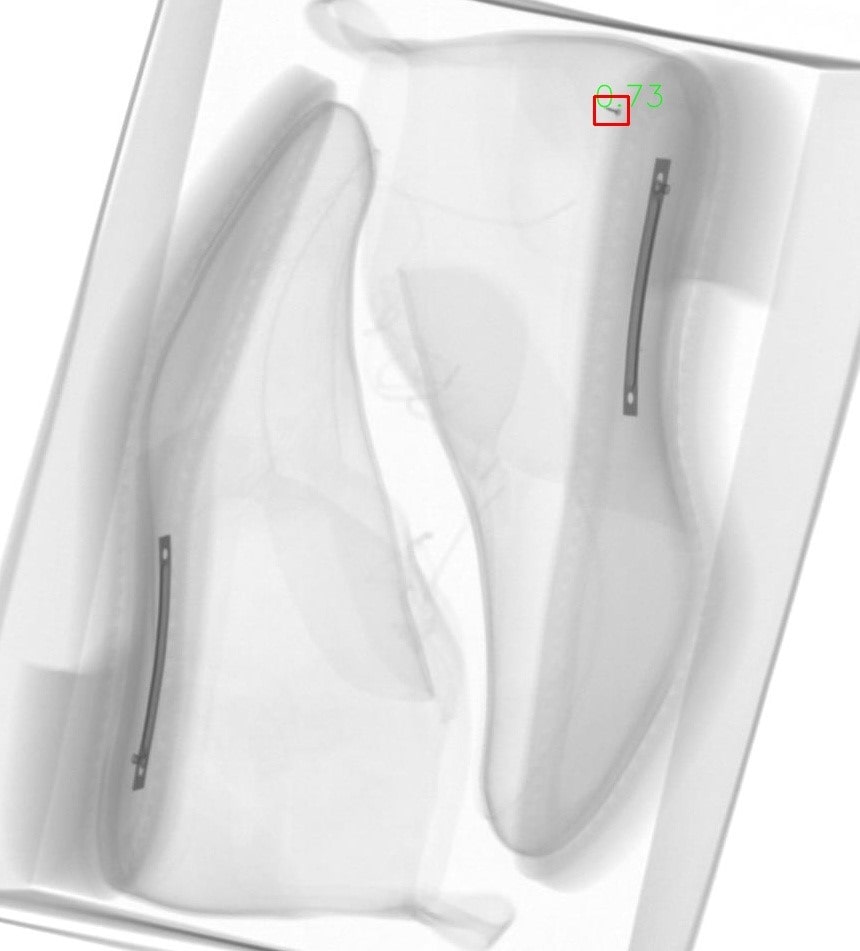
The images of different categories of shoes and relative annotations gathered in the first part of this project will be used to implement and train deep learning models for the identification of the position of metallic elements such as nails within each pair of shoes. The annotation of such images is possible thanks to an annotation tool developed during this project that helps the human annotator suggesting possible labels for the metallic elements. The developed deep learning models aim to identify the border of the shoes, predicting the size and number of shoes within each box. Furthermore, the models will provide detailed information of the metallic elements included in the shoes, identifying the type of element and its position within each shoe. This information will be used to discriminate metallic elements that affect the integrity of the shoes, considered as defects (i.e., located in a wrong area of the shoes, outside the heel area). The models parameters will be tuned to achieve 95% of accuracy in the detection of defects while minimizing the false positive rate (i.e., considering there is a defect in a shoe that has no defects) up to 3%. The final release of the deep learning models will be integrated in the current available Brustia software for the X ray image analysis, enabling the analysis of the acquired X-ray image with the pipeline of the YK software. The analysis prediction will be read by the Brustia software for further defects management, while the original image and the relative annotation will be stored online, enlarging the dataset and enabling the re-train of the deep learning models with new examples The software will be installed in three Brustia’s clients for testing purposes.
Dissemination
ComoNExT Website
https://www.comonext.it/il-progetto-proofer-e-stato-selezionato-dal-bando-dih/
https://www.linkedin.com/feed/update/urn:li:activity:6988792203804315648
https://www.linkedin.com/feed/update/urn:li:activity:7003387702788157440
https://www.instagram.com/p/Cj7jh72oo0Z
https://www.instagram.com/p/CljQtqItulj
The two Instagram posts are also on ComoNExT Facebook account.
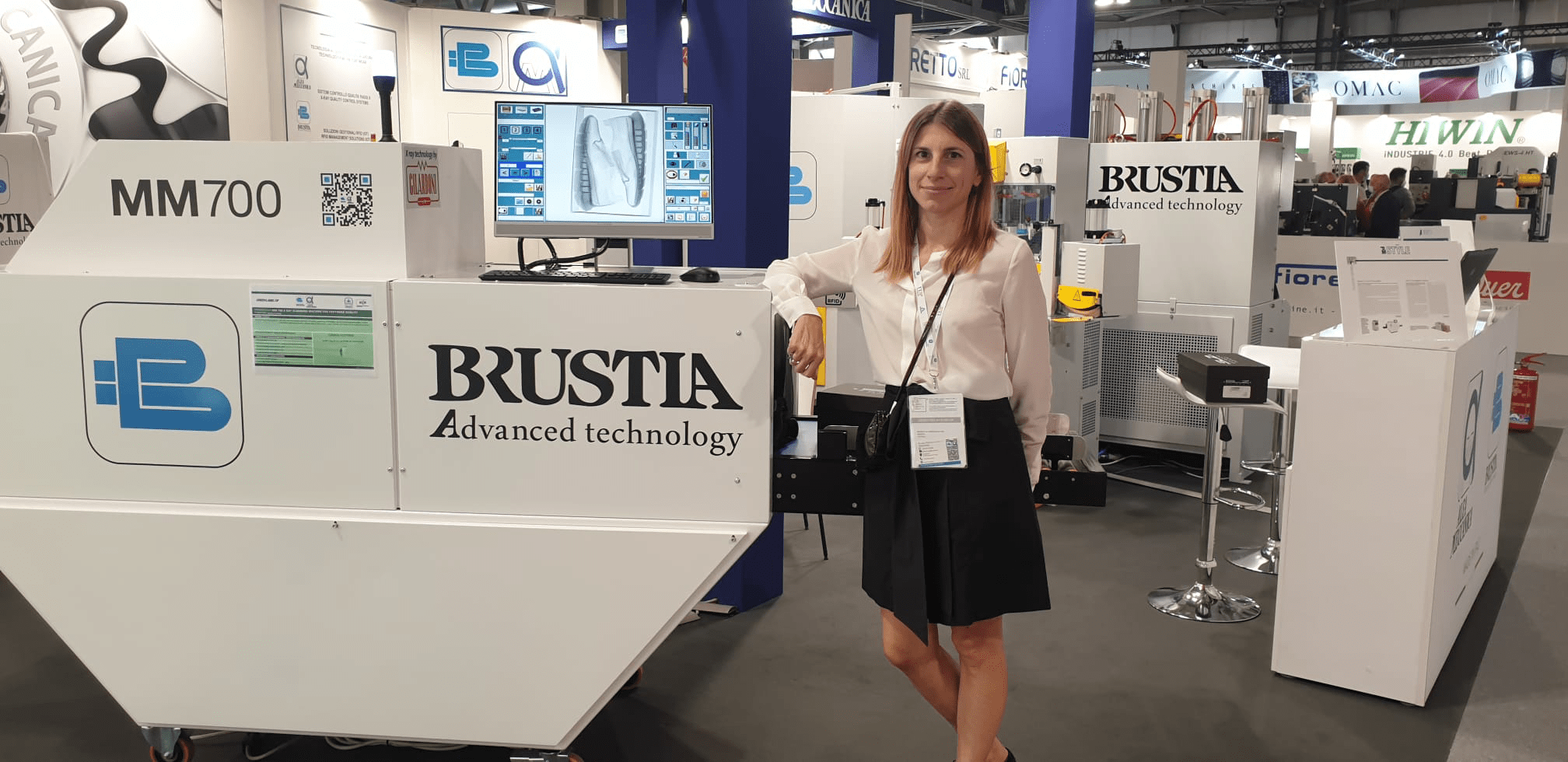